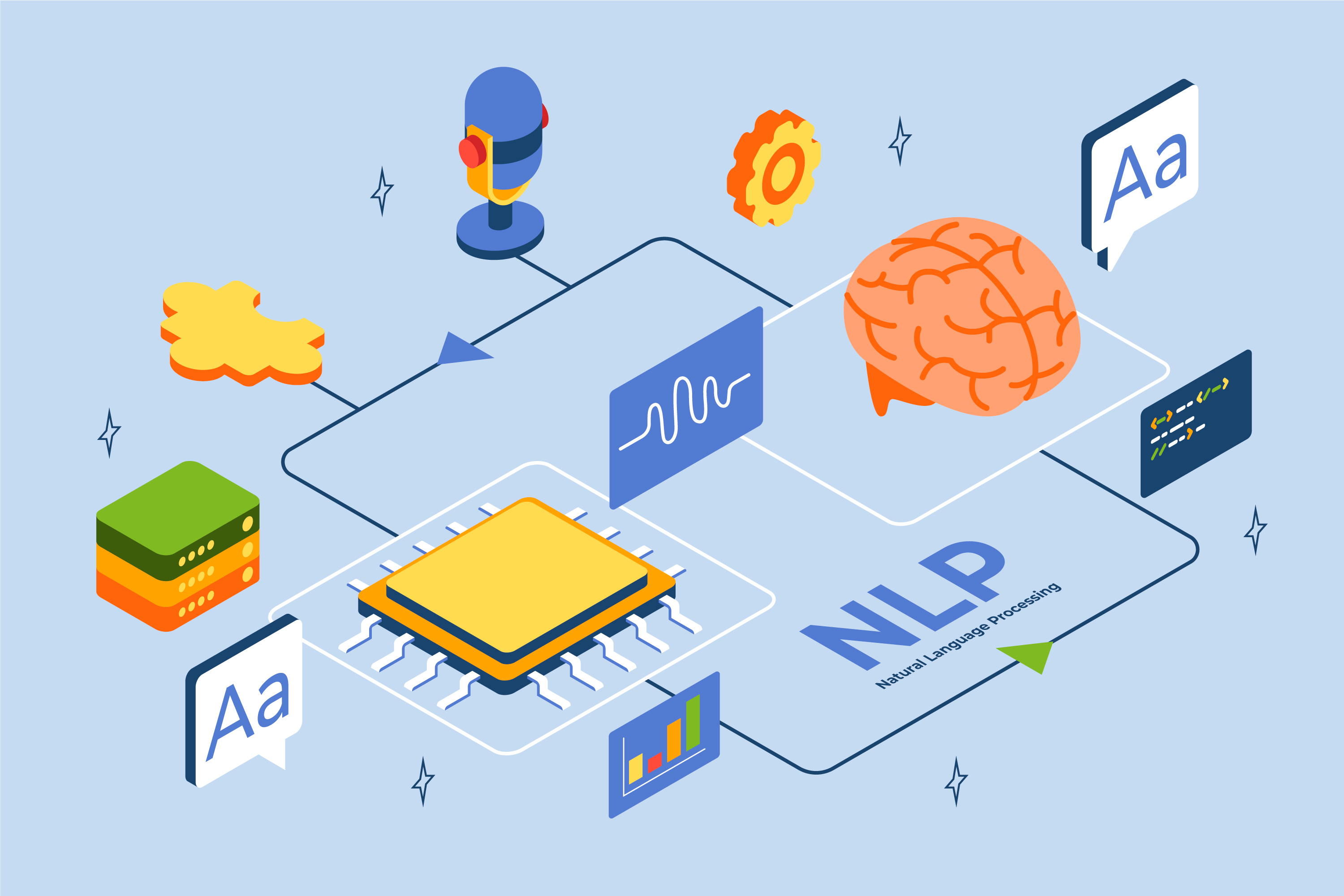
Best practices for your next AI project
Applying AI technology to your company’s business can be a daunting task. We have collected some best practices that will help mitigate risk and show you how to successfully manage implementing AI in your organization.
Define SMART Objectives
Make sure your business objectives are SMART – specific, measurable, actionable, relevant and time-bound. Balance these against your organization’s implementation readiness. Realistic and well-defined business objectives make it easier it to measure success and get stakeholder buy-in.
Start with a Pilot project now
Paralysis by analysis can be a real problem when a technology like AI could be applicable to so many use cases in your organization. You can avoid this by picking a pilot project with clear objectives, measurable benefits and a reasonable timeline. Don’t try to solve for all potential use cases to start. Pick one with reasonably low costs, straightforward implementation and measurable benefits.
- Ensure that the AI pilot demonstrates clear benefits or improvements over existing processes. This could be in terms of efficiency, accuracy, cost savings, or quality.
- Determine the resources (financial, technical, and human) needed for the pilot project, and assess whether your company can support these requirements.
- Gain support from key stakeholders within your company, including executives, IT personnel, and relevant department heads.
- Evaluate how easily the AI solution can be scaled from the pilot project to wider implementation across different departments or functions.
- Budget and plan for training and onboarding or stakeholders and end users from the beginning. Some learning and development companies like Metrix have AI implementation departments that are tailored to address this specific need. Poor training and onboarding of stakeholders and end-users is one of the leading causes of sub-optimal implementation of AI.
Pilot project Selection
Choose a specific use case where AI can provide tangible benefits and where the pilot project can demonstrate its feasibility and value. At Papercurve we have AI solutions for many potential use cases that could be beneficial to your organization and can integrate seamlessly with existing software systems.
References powered by Paige AI uses Natural Language Processing (NLP) to understand selected text and suggest relevant references based on previous usage.
-p-800.png)
Product Claims powered by Paige AI uses NLP to find approved product claims that most closely match selected text and highlights any variations that may be problematic from a regulatory perspective.
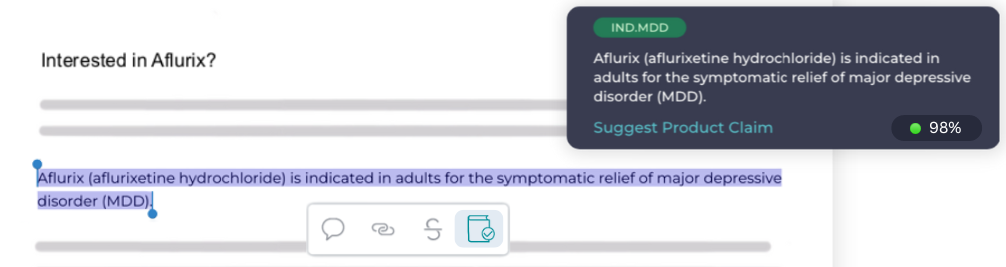
Generative AI for compliant regulated content creation
Papercurve Office powered by Paige AI allows users to create content in Office files in the browser and have Paige suggest approved wording and reference annotations as you type in real time.
.gif)
Evaluation Framework
Develop a framework to evaluate the success of the pilot project based on predefined metrics and criteria. This should include both quantitative (e.g., performance metrics) and qualitative (e.g., user feedback) measures.
Timeline and Milestones
Establish a realistic timeline with achievable milestones for the pilot project. This helps in monitoring progress and making necessary adjustments along the way.
Budget Allocation
Allocate sufficient budget for the pilot project, considering costs associated with AI development, data acquisition, infrastructure setup, and personnel.
Access to Quality Data
Good data is a strict requirement for all successful AI projects. Ensure access to high-quality, diverse datasets that are relevant to your objectives. Using your company’s own data is the best place to start.
- What if your organization uses Veeva Vault to store its data? Veeva confirmed in its Q1 FY2025 earnings remarks on May 30, 2024 that “We don’t have plans to develop or acquire GenAI solutions today” but it does provide GenAI companies like Papercurve access to Vault data via its new Vault Direct Data API.
- What if your data needs to be better organized or includes duplicates?
AI is a powerful tool for organizing large amounts of data efficiently. Here are some examples of how AI tools can help organize your information:
Document Classification: AI can classify documents into categories based on their content. This can be done using techniques like natural language processing (NLP) to analyze the text and assign relevant tags, metadata or categories.
Metadata Extraction: AI can extract key metadata from documents such as dates, authors, titles, and key phrases. This metadata can then be used for sorting and organizing documents.
Search and Retrieval: AI-powered search engines can help users quickly find documents based on keywords, phrases, or even concepts within the text, making it easier to locate specific information.
Summarization: AI can generate summaries of documents, which can be useful for quickly understanding the main points without having to read through hundreds of pages of text.
Document Similarity: AI algorithms can measure similarity between documents, helping to group related documents together and flag duplicates for removal.
Automation of Workflows: AI can automate repetitive tasks such as sorting, tagging, and organizing documents based on predefined rules or learned patterns.
Regulatory and Ethical Considerations
Understand and comply with regulatory requirements (e.g., FDA guidelines in the US) and ethical standards when dealing with patient data and AI algorithms. Develop AI solutions that are transparent, explainable, and accountable. Organizations like the Responsible AI Institute can be an be invaluable resources in helping your team in this area.
Monitor Performance and Impact
Establish metrics to measure the performance and impact of AI solutions on key outcomes like productivity and cost savings. Keep in mind that the single most important metric to monitor is end user adoption. Without good end user onboarding and adoption, the project will not achieve it full potential. Use insights from these metrics to refine strategies and improve future projects.
By following these considerations and requirements, your company can effectively plan and execute an AI pilot project that serves as a robust foundation for a wider roll out of AI initiatives in the future. This approach helps mitigate risks, demonstrate value, and ensure alignment with strategic objectives before scaling across the organization.
The most important thing to remember with your AI pilot project is to start the ball rolling now. Contact Papercurve here for a free consultation on how to start the process of applying AI to your business objectives.